Improving the efficiency of cascading failure analysis in power systems by surrogate modelling
Description
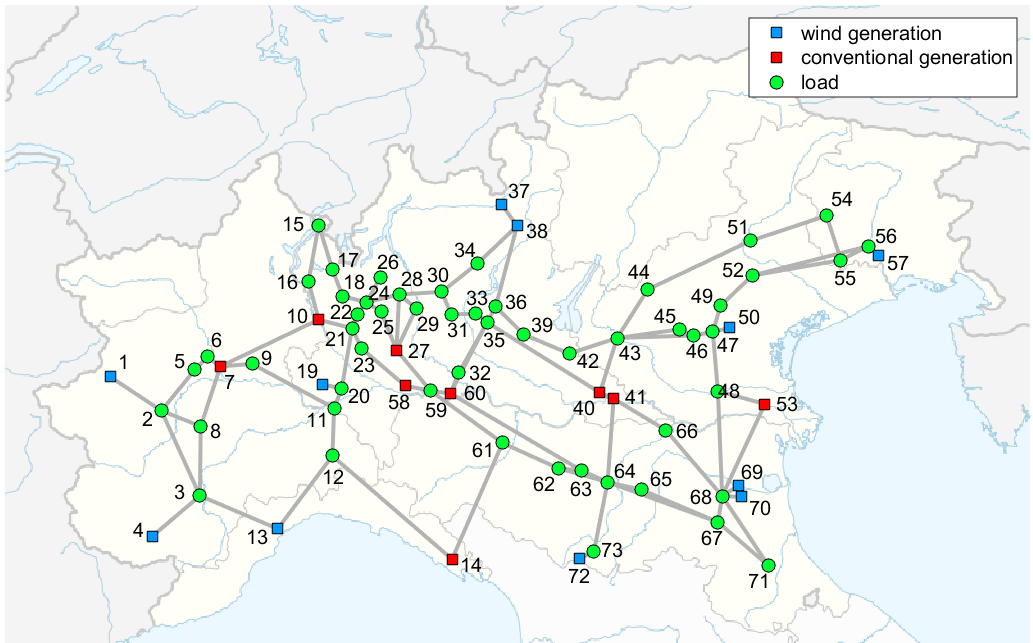
Power systems are complex infrastructures that transform and transport energy produced from distributed sources. Several environmental and operating conditions have an influence on such systems, possibly leading to a non-complete satisfaction of the energy demand and, in more severe cases, to blackouts. Many of these factors are uncertain, thus leading to an uncertain output of the system. Physically detailed computational models of these systems provide an accurate prediction of the response to a specific input, but are computationally expensive to run. Thus, predicting the statistics of the response by resampling from the input distribution (Monte-Carlo approach) is typically not affordable. This collaboration project investigates the relevance of inexpensive surrogate models, such as polynomial chaos expansions and Kriging, to approximate the original model and thus to enable uncertainty quantification of its output.