Active learning methods for the probabilistic robust design of control systems for cyber-physical systems
Description

Cyber-physical empirical methods consist in partitioning a dynamical system under study into a set of physical and numerical substructures that interact in real-time through a control system. In this project, we investigate the fidelity and robustness of such methods in the presence of noise and other artefacts that can affect the control systems. In practice, fidelity is jeopardized by uncertain and heterogeneous artefacts originating from the control system, such as actuator dynamics, time delays and measurement noise. We developed and assessed a computationally efficient method, based on surrogate modelling and active learning techniques, that can (1) verify that a cyber-physical empirical setup achieves probabilistic robust fidelity, and (2) derive fidelity bounds, which translate to absolute requirements to the control system. The developed methodology is then applied to the study of a complex problem, namely the active truncation of slender marine structures, in which the substructures’ dynamics cannot be described by an analytic solution.
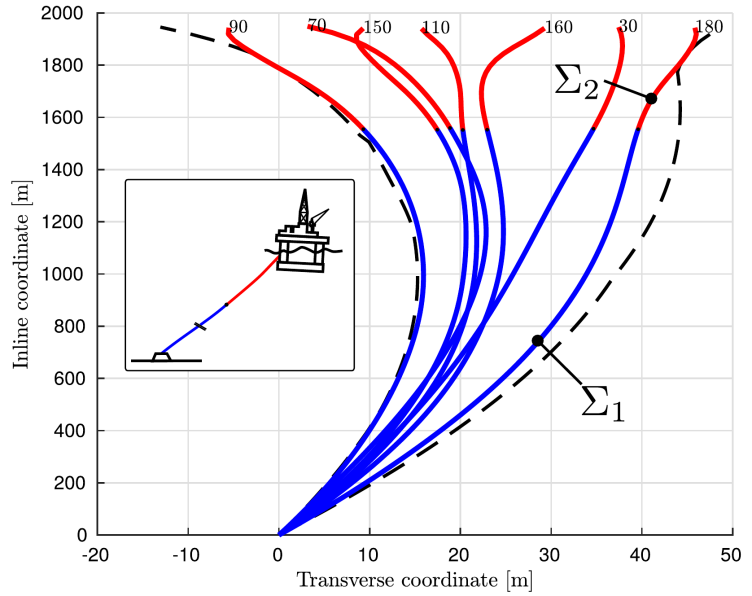
Cyber-physische empirische Methoden teilen ein dynamisches System in physische und numerische Substrukturen auf, die in Echtzeit durch ein Steuerungssystem miteinander interagieren. In diesem Projekt untersuchen wir die Genauigkeit und Robustheit solcher Methoden, wenn Störungen und andere Artefakte die Steuerungssysteme beeinflussen. In der Praxis wird die Genauigkeit durch ungewisse heterogene Artefakte beeinträchtigt, die durch das Steuerungssystem selbst verursacht werden, wie z.B. Aktuatordynamik, zeitliche Verzögerungen und Messungenauigkeiten. Wir haben eine recheneffiziente Methode entwickelt und getestet, die Ersatzmodelle und aktive maschinelle Lernmethoden verwendet. Diese Methode kann (1) nachweisen, dass ein cyber-physisches empirisches Setup probabilistisch robuste Genauigkeit erreicht, und (2) Genauigkeitsschranken herleiten, die absoluten Anforderungen an das Steuerungssystem entsprechen. Die entwickelte Methodik wurde auf das anspruchsvolle Problem der aktiven Verkürzung von schlanken marinen Strukturen angewandt, bei dem die Dynamik der Substrukturen nicht analytisch beschrieben werden kann.
References