Generalized lambda distributions to surrogate stochastic simulators
Principal investigators: X. Zhu
Description
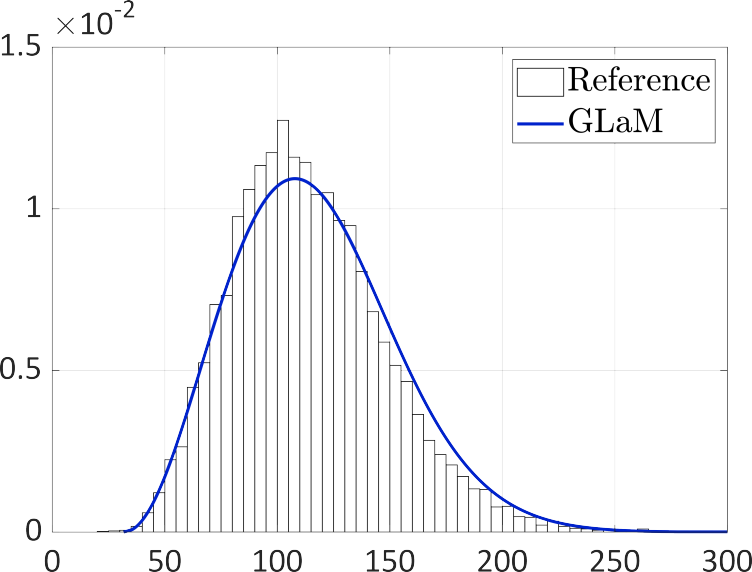
As opposed to deterministic simulators, stochastic simulators contain intrinsic randomness and produce different values of the model output when run several times with the same input parameters. Therefore, the output of a stochastic simulator is a random variable, and each model evaluation provides a single realization of the latter.
Due to their random nature, stochastic simulators need to be repeatedly evaluated for a given set of input parameters (called replications) to fully characterize the corresponding model response. When investigating such analysis for various input values, the number of model evaluations quickly becomes intractable for expensive computational models.
In this project, we develop surrogate models to emulate the response probability distribution of stochastic simulators. We propose using the flexible generalized lambda distributions to approximate the response distribution. The dependence of the distribution parameters on the input is represented by polynomial chaos expansions (external page Zhu and Sudret, 2020). The developed method (external page Zhu and Sudret, 2021) combines several statistical learning methods and features no need for replications. Studies show that the proposed method outperforms state-of-the-art nonparametric kernel estimators and yields accurate estimates in the global sensitivity analysis for stochastic simulators.
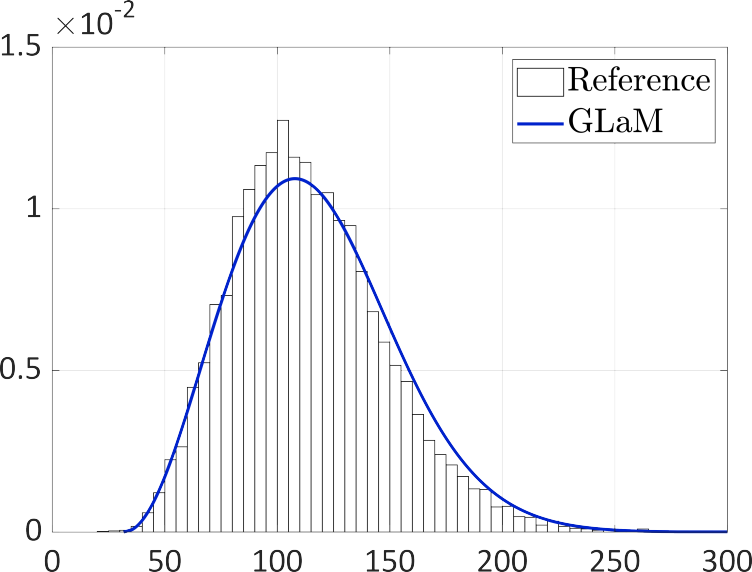
Anders als deterministische Simulatoren besitzen stochastische Simulatoren intrinsische Zufälligkeit und liefern verschiedene Outputwerte, selbst wenn sie mehrmals mit den gleichen Inputparametern ausgeführt werden. Daher ist der Output eines stochastischen Simulators eine Zufallsvariable, und jede Modellauswertung entspricht einer einzigen Realisierung derselben.
Wegen ihrer Zufallsnatur müssen stochastische Simulatoren für jeden Satz von Inputparametern mehrmals ausgewertet werden („Replikationen“), um die Modellantwort ausreichend zu charakterisieren. Wenn eine solche Analyse für viele Inputparametervektoren durchgeführt werden muss, erreicht man schnell eine unrealistisch hohe Anzahl teurer Modellauswertungen.
In diesem Project entwickeln wir Ersatzmodelle, um die Wahrscheinlichkeits-verteilung des Outputs von stochastischen Simulatoren nachzubilden. Wir verwenden dafür die flexiblen verallgemeinerten Lambda-Verteilungen. Die Abhängigkeit der Verteilungs-parameter vom Input wird durch polynomial chaos expansions modelliert (external page Zhu und Sudret, 2020). Unsere Methode (external page Zhu und Sudret, 2021) kombiniert mehrere Methoden des statistischen Lernens und benötigt keine Replikationen. Unsere Experimente zeigen, dass sie nichtparametrische Kerndichteschätzer übertrifft und genaue Schätzwerte für die globale Sensitivitätsanalyse von stochastischen Simulatoren liefert.