Active learning for structural reliability analysis
Principal investigators: M. Moustapha, S.Marelli
Description
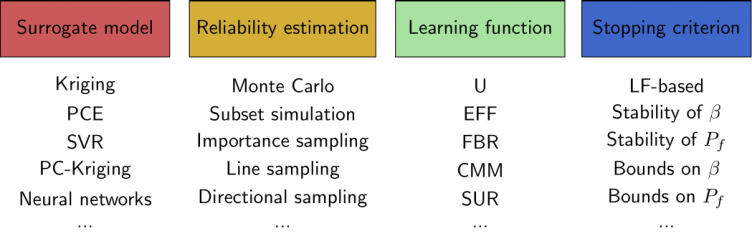
Structural reliability analysis is essential in the assessment of the safety of structures throughout their entire life cycle. Practically, the analyst computes a failure probability by accounting for various uncertainties related either to the inherent variability of the structural properties or of their operational conditions. The underlying mathematical problem is cumbersome as it requires solving an integration problem over an implicitly defined domain. Numerical integration through simulation methods (e.g. Monte Carlo or subset simulation) provide accurate solutions but at the expense of an extremely high computational cost.
Active learning, a process by which the computational model describing the system behaviour is replaced by an inexpensive surrogate trained on an actively enriched sample set, has been the subject of a wide body of research since its introduction in structural reliability.
In this project, we conducted a thorough survey of active learning reliability methods, which showed that most existing contributions fit into a generalized framework that combines ingredients from four different components (external pageMoustapha et al, 2022call_made). This framework was implemented as a new module in UQLab, a Matlab framework for uncertainty quantification. Using this module, we conducted an extensive benchmark comprising more than 12,000 reliability analyses. The results of this benchmark led us to establish general user-oriented recommendations. The latter were then used to participate in the TNO black-box reliability challenge, where our submitted solutions were the most accurate and efficient compared to those submitted by the other participants (external pageRozsas and Slobbe, 2019call_made).
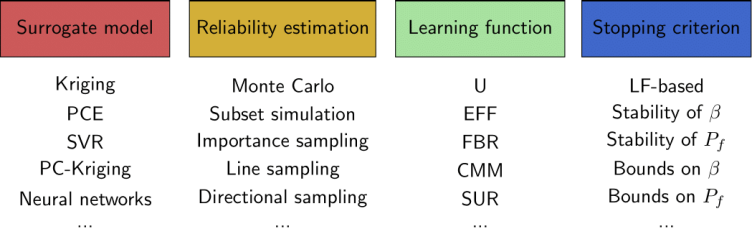
Die Zuverlässigkeitsanalyse ist wesentlich für die Beurteilung der Sicherheit von Gebäuden während des gesamten Lebenszyklus. In der Praxis berechnet man eine Versagenswahrscheinlichkeit, indem verschiedene Ungewissheiten berücksichtigt werden, die entweder mit der Variabilität der Gebäudeeigenschaften oder ihrer Betriebsbedingungen zusammenhängen. Das zugrundeliegende mathematische Problem ist herausfordernd, da ein Integrationsproblem über eine implizit definierte Domäne gelöst werden muss. Die numerische Integration mit Simulationsmethoden (z. B. Monte Carlo oder subset simulation) liefert genaue Lösungen, benötigt jedoch einen extrem hohen Rechenaufwand.
Aktives Lernen bei Zuverlässigkeitsanalysen ist Gegenstand vieler Forschungsarbeiten, dabei wird das Systemmodell durch ein kostengünstiges Ersatzmodell ersetzt, welches mit einer aktiv angereicherten Stichprobe trainiert wird.
In diesem Projekt haben wir eine Analyse der bestehenden Methoden zum aktiven Lernen bei Zuverlässigkeitsanalysen durchgeführt, die gezeigt hat, dass die meisten Methoden in ein allgemeines Framework passen (external pageMoustapha et al., 2022call_made). Dieses Framework wurde als neues Modul in UQLab implementiert, einer Matlab-Software zur Quantifizierung der Ungewissheiten. Mit diesem Modul haben wir einen umfangreichen Vergleichstest berechnet und anhand der Ergebnisse allgemeine, benutzerorientierte Empfehlungen erstellt. Diese wurden für die Teilnahme an der TNO black-box-reliability challenge verwendet, bei der unsere Ergebnisse im Vergleich zu anderen Teilnehmern am genauesten und effizientesten waren (external pageRozsas and Slobbe, 2019call_made).
References
Rozsas, A. and Slobbe, A. (2019). Repository and black-box reliability challenge 2019, external pagehttps://gitlab.com/rozsasarpi/rprepo/call_made Accessed: 2023-03-23.