New paper published in Structural Safety
Anderson V. Pires, M. Moustapha, S. Marelli, and B. Sudret publish a new paper showcasing active learning techniques for reliability analysis of noisy systems.
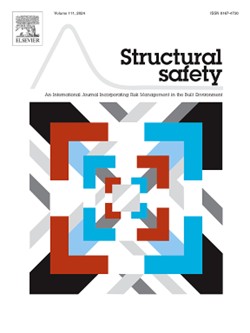
In our work entitled "Reliability analysis for data-driven noisy models using active learning," we propose a novel methodology for performing reliability analysis on noise-corrupted systems. To achieve this, we utilize regression-based surrogate models as denoisers. Specifically, we demonstrate that Gaussian process regression is particularly effective for this purpose. Furthermore, to enhance computational efficiency, we introduce noise-aware learning functions. The proposed methodology is validated through benchmark examples and a realistic structural frame, showcasing its robustness in handling high-dimensional inputs, complex failure scenarios, and significant noise levels.
For more information, please visit external page this external page link for the publication and this link for the associated internal report.